Project 3 bonus: More Fake News! 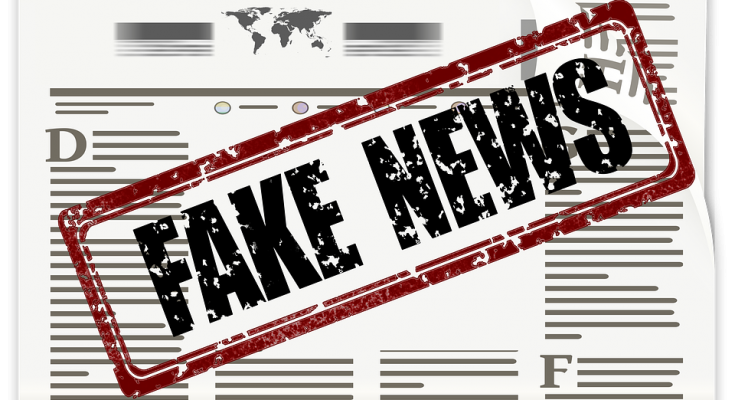
For the Project 3 bonus, you will keep working on the Project 3 task, and try to make a better “fake news” detector.
Part 1 (up to 1.5 marks toward your final grade)
Part 1 (a)
Build a better “fake news’’ detector. You may use any other data source (for example, see here), and any other model or algorithm. Report on what you did, on how much you improved the performance on the Project 3 dataset we provided compared to the Naive Bayes, Decision Tree, and Logistic Regression models. Explain how you evaluated your improvements.
Note that results you obtained on the Project 3 dataset we provided may not be representative of the performance of your model in general (which we will test in Part 2). Specifically, the “real news’’ headlines contained news from a single Australian data source. If you evaluated your model performance in other ways, clearly explain what you did and why.
Your mark will depend on how technically interesting the work is and the quality of your analysis.
Part 1 (b)
In Project 3, you displayed lists of keywords to explain how your classifier works. For your new method, produce an appropriate interpretation for how it works.
Part 2 (up to 1 marks toward your final grade)
For this part, we will hold a “fake news” detection competition. We will compile a test set of recent news headlines, and hand-label them to be “real” or “fake”. The format of the test set will be the same as the Project 3 test set.
Submit a script that implements the method that you used in Part 1 that can be run using one of the following commands in the CS Teaching Labs:
python classify_news.py headlines.txt
or
python3 classify_news.py headlines.txt
The first line in your file must be either #!/usr/bin/python or #!/usr/bin/python3, depending on which version of Python you’re using.
The file headlines.txt will contain n lines, with one headline per line. Your script should output n lines, with 0 corresponding to “fake”/bs/etc., and 1 corresponding to real.
Example input:
white house says exemptions possible from trump trade tariffs obama signs executive order banning the pledge of allegiance in schools nationwide trump says russia had no impact on 2016 election votes
Example output:
1 0 1
Your script should take under 1 minute to run for 100 examples.
Please submit the only one set of weights for Part 1 and Part 2 (but you should fit multiple models and explore how they affect the performance.) Be especially careful with your classify_news.py file — it must run as is, without us having to fix it.
Prizes: 1.0 marks for best performance, 0.95 marks for second place, 0.9 marks for third place, 0.6 marks for honourable mentions.
Please make sure we can run your script on the CS Teaching Labs as is.
What to submit
The project should be implemented using Python 2 or 3, and should be runnable on the CS Teaching Labs computers. Your report should be in PDF format. You should use LaTeX to generate the report, and submit the .tex file as well. A sample template is on the course website. You will submit at least the following files: classify_news.py
, fakebonus.py
, fakebonus.tex
, and fakebonus.pdf
. You may submit more files as well. You may submit ipynb
files in place of fakebonus.py
, but not in place of classify_news.py
. You might like to submit a set of weights. If your weight file size exceeds the MarkUs file size limit, you may include a script to download one weights file (under 1GB) from the internet. You must include a SHA-256 hash of the weights file with your submission.
Reproducibility counts! We should be able to obtain all the graphs and figures in your report by running your code. The only exception is that you may pre-download the images (what and how you did that, including the code you used to download the images, should be included in your submission.) Submissions that are not reproducible will not receive full marks. If your graphs/reported numbers cannot be reproduced by running the code, you may be docked up to 20%. (Of course, if the code is simply incomplete, you may lose even more.) Suggestion: if you are using randomness anywhere, use numpy.random.seed()
.
You must use LaTeX to generate the report. LaTeX is the tool used to generate virtually all technical reports and research papers in machine learning, and students report that after they get used to writing reports in LaTeX, they start using LaTeX for all their course reports. In addition, using LaTeX facilitates the production of reproducible results.
Available code
You are free to use any of the code available from the course website, and anything else runnable on CS Teaching Lab computers. Clearly identify what code is yours and what code you obtained from elsewhere.
Readability
Readability counts! If your code isn’t readable or your report doesn’t make sense, they are not that useful. In addition, the TA can’t read them. You will lose marks for those things.
Academic integrity
It is perfectly fine to discuss general ideas with other people, if you acknowledge ideas in your report that are not your own. However, you must not look at other people’s code, or show your code to other people, and you must not look at other people’s reports and derivations, or show your report and derivations to other people. All of those things are academic offences.